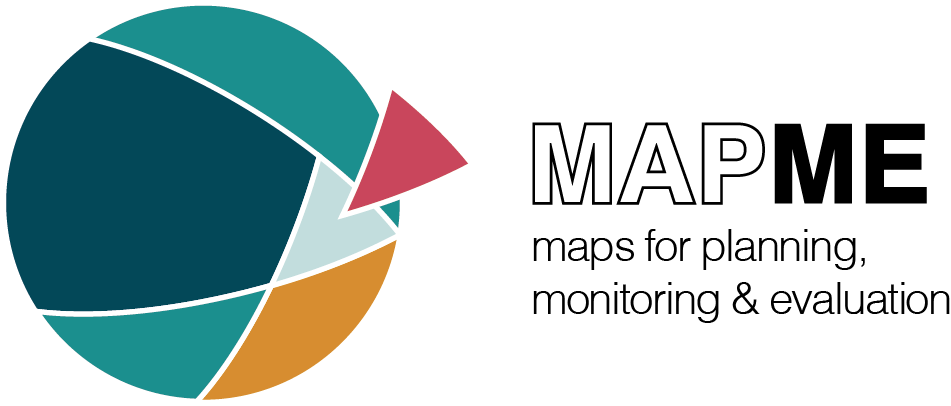
Calculates a user defined set of landscape fragmentation indices for patch
types identified in a raster
*Object.
FragStatsCalc.Rd
Calculates a user defined set of landscape fragmentation indices for patch
types identified in a raster
*Object.
Usage
FragStatsCalc(
inputRasterFiles = NULL,
latlon = NULL,
polyName = NULL,
studysite = NULL,
FragStats = "all",
ncores = 1,
saveCSV = FALSE
)
Arguments
- inputRasterFiles
A
raster
-Object. Fragmentation statistics area calculated seperatly for every layer.- latlon
logical
: Indicates whether or not theinputForestMap
object is based on a geographic coordinate system or is projected to a planar coordinate system. In the former case, area is approximated byraster::area
.- polyName
charachter
of length 1. Indicates the column in the data frame of thestudysite
object to uniquely identify the features of interest. The function will fail if there is no unique identification of the polygons- studysite
An object of type
sf
with a given number of polygons defining the areas of interest. Forest area statistics will be calculated for features in thestudysite
object.- FragStats
Character vector of forest fragmentation parameters to be calculated. Currently available statistics are:
n.patches the number of patches of a particular patch type or in a class
total.area the sum of the areas (m2) of all patches of the corresponding patch type
prop.landscape the proportion of the total lanscape represented by this class
patch.density the numbers of patches of the corresponding patch type divided by total landscape area
total.edge the total edge length of a particular patch type
edge.density edge length on a per unit area basis that facilitates comparison among landscapes of varying size
mean.patch.area average area of patches
mean.frac.dim.index mean of fractal dimension index
lanscape.division.index based on the cumulative patch area distribution and is interpreted as the probability that two randomly chosen pixels in the landscape are not situated in the same patch
patch.cohesion.index measures the physical connectedness of the corresponding patch type
landscape.shape.index a standardized measure of total edge or edge density that adjusts for the size of the landscape
largest.patch.index largest patch index quantifies the percentage of total landscape area comprised by the largest patch
sd.patch.areastandard deviation of patch areas
min.patch.area the minimum patch area of the total patch areas
max.patch.area the maximum patch area of the total patch areas
perimeter.area.frac.dim perimeter-area fractal dimension equals 2 divided by the slope of regression line obtained by regressing the logarithm of patch area (m2) against the logarithm of patch perimeter (m)
mean.perim.area.ratio the mean of the ratio patch perimeter. The perimeter-area ratio is equal to the ratio of the patch perimeter (m) to area (m2)
sd.perim.area.ratio standard deviation of the ratio patch perimeter
max.perim.area.ratio maximum perimeter area ratio
mean.shape.index mean of shape index
sd.shape.index standard deviation of shape index
min.shape.index the minimum shape index
max.shape.index the maximum shape index
sd.frac.dim.index standard deviation of fractal dimension index
min.frac.dim.index the minimum fractal dimension index
max.frac.dim.index the maximum fractal dimension index
total.core.areathe sum of the core areas of the patches (m2)
prop.landscape.core proportional landscape core
mean.patch.core.area mean patch core area
sd.patch.core.area standard deviation of patch core area
min.patch.core.area the minimum patch core area
max.patch.core.area the maximum patch core area
prop.like.adjacencies calculated from the adjacency matrix, which shows the frequency with which different pairs of patch types (including like adjacencies between the same patch type) appear side-by-side on the map (measures the degree of aggregation of patch types)
aggregation.index computed simply as an area-weighted mean class aggregation index, where each class is weighted by its proportional area in the landscape
izesplitting.index based on the cumulative patch area distribution and is interpreted as the effective mesh number, or number of patches with a constant patch size when the landscape is subdivided into S patches, where S is the value of the splitting index
effective.mesh.size equals 1 divided by the total landscape area (m2) multiplied by the sum of patch area (m2) squared, summed across all patches in the landscape
- ncores
The number of cores to use, i.e. at most how many child processes will be run simultaneously.
- saveCSV
Default is
FALSE
. You can specify a directory on your local machine where the results area saved in.csv
format. The features will be identified by the column specified inpolyName
.
Value
An sf
-object with it's dataframe ammended by the specified
fragmentation statistics with one column for every layer
in inputRasterFiles
representing observation years. Additionally trend
statistics and the difference between the first and last observation
are automatically calculated for every single parameter based on
trend:sens.slope
.
Author
Darius Görgen (MapTailor Geospatial Consulting GbR) info@maptailor.net
Maintainer: MAPME-Initiative contact@mapme-initiative.org
Contact Person: Dr. Johannes Schielein
Copyright: MAPME-Initiative
License: GPL-3
Examples
library(sf)
library(raster)
library(mapme.forest)
aoi = st_read(system.file("extdata", "aoi_polys.gpkg", package = "mapme.forest"))
#> Reading layer `aoi_polys' from data source
#> `/home/runner/work/_temp/Library/mapme.forest/extdata/aoi_polys.gpkg'
#> using driver `GPKG'
#> Simple feature collection with 8 features and 1 field
#> Geometry type: POLYGON
#> Dimension: XY
#> Bounding box: xmin: 107.1291 ymin: 16.59741 xmax: 107.2502 ymax: 16.6916
#> Geodetic CRS: WGS 84
yearlyRaster = stack(system.file("extdata", "pkgTest_yearlyCover.tif",
package = "mapme.forest"))
names(yearlyRaster) = paste("y_", as.character(2000:2018))
result = FragStatsCalc(inputRasterFiles = yearlyRaster,
studysite = aoi[1,],
FragStats = c("n.patches", "total.area",
"prop.landscape", "patch.density"),
latlon = TRUE,
polyName = "id",
ncores = 1,
saveCSV = FALSE)
str(result)
#> Classes ‘sf’ and 'data.frame': 1 obs. of 90 variables:
#> $ id : int 1
#> $ n.patches_Dif : num 15
#> $ n.patches_Sig : num 0.019
#> $ n.patches_Slope : num 0.938
#> $ n.patches_y_.2000 : num 1
#> $ n.patches_y_.2001 : num 1
#> $ n.patches_y_.2002 : num 1
#> $ n.patches_y_.2003 : num 1
#> $ n.patches_y_.2004 : num 1
#> $ n.patches_y_.2005 : num 5
#> $ n.patches_y_.2006 : num 16
#> $ n.patches_y_.2007 : num 16
#> $ n.patches_y_.2008 : num 19
#> $ n.patches_y_.2009 : num 20
#> $ n.patches_y_.2010 : num 20
#> $ n.patches_y_.2011 : num 20
#> $ n.patches_y_.2012 : num 15
#> $ n.patches_y_.2013 : num 17
#> $ n.patches_y_.2014 : num 16
#> $ n.patches_y_.2015 : num 15
#> $ n.patches_y_.2016 : num 16
#> $ n.patches_y_.2017 : num 16
#> $ n.patches_y_.2018 : num 16
#> $ patch.density_Dif : num 1.88e-05
#> $ patch.density_Sig : num 0.019
#> $ patch.density_Slope : num 1.18e-06
#> $ patch.density_y_.2000 : num 1.26e-06
#> $ patch.density_y_.2001 : num 1.26e-06
#> $ patch.density_y_.2002 : num 1.26e-06
#> $ patch.density_y_.2003 : num 1.26e-06
#> $ patch.density_y_.2004 : num 1.26e-06
#> $ patch.density_y_.2005 : num 6.28e-06
#> $ patch.density_y_.2006 : num 2.01e-05
#> $ patch.density_y_.2007 : num 2.01e-05
#> $ patch.density_y_.2008 : num 2.39e-05
#> $ patch.density_y_.2009 : num 2.51e-05
#> $ patch.density_y_.2010 : num 2.51e-05
#> $ patch.density_y_.2011 : num 2.51e-05
#> $ patch.density_y_.2012 : num 1.88e-05
#> $ patch.density_y_.2013 : num 2.14e-05
#> $ patch.density_y_.2014 : num 2.01e-05
#> $ patch.density_y_.2015 : num 1.88e-05
#> $ patch.density_y_.2016 : num 2.01e-05
#> $ patch.density_y_.2017 : num 2.01e-05
#> $ patch.density_y_.2018 : num 2.01e-05
#> $ prop.landscape_Dif : num -0.942
#> $ prop.landscape_Sig : num 2.84e-08
#> $ prop.landscape_Slope : num -0.0513
#> $ prop.landscape_y_.2000: num 0.991
#> $ prop.landscape_y_.2001: num 0.991
#> $ prop.landscape_y_.2002: num 0.991
#> $ prop.landscape_y_.2003: num 0.991
#> $ prop.landscape_y_.2004: num 0.99
#> $ prop.landscape_y_.2005: num 0.699
#> $ prop.landscape_y_.2006: num 0.247
#> $ prop.landscape_y_.2007: num 0.247
#> $ prop.landscape_y_.2008: num 0.213
#> $ prop.landscape_y_.2009: num 0.207
#> $ prop.landscape_y_.2010: num 0.207
#> $ prop.landscape_y_.2011: num 0.207
#> $ prop.landscape_y_.2012: num 0.127
#> $ prop.landscape_y_.2013: num 0.0671
#> $ prop.landscape_y_.2014: num 0.0531
#> $ prop.landscape_y_.2015: num 0.0494
#> $ prop.landscape_y_.2016: num 0.0485
#> $ prop.landscape_y_.2017: num 0.0485
#> $ prop.landscape_y_.2018: num 0.0485
#> $ total.area_Dif : num -750111
#> $ total.area_Sig : num 2.84e-08
#> $ total.area_Slope : num -40807
#> $ total.area_y_.2000 : num 788692
#> $ total.area_y_.2001 : num 788692
#> $ total.area_y_.2002 : num 788692
#> $ total.area_y_.2003 : num 788692
#> $ total.area_y_.2004 : num 787950
#> $ total.area_y_.2005 : num 556462
#> $ total.area_y_.2006 : num 196616
#> $ total.area_y_.2007 : num 196616
#> $ total.area_y_.2008 : num 169906
#> $ total.area_y_.2009 : num 164712
#> $ total.area_y_.2010 : num 164712
#> $ total.area_y_.2011 : num 164712
#> $ total.area_y_.2012 : num 100905
#> $ total.area_y_.2013 : num 53420
#> $ total.area_y_.2014 : num 42291
#> $ total.area_y_.2015 : num 39323
#> $ total.area_y_.2016 : num 38581
#> $ total.area_y_.2017 : num 38581
#> $ total.area_y_.2018 : num 38581
#> $ geometry :sfc_POLYGON of length 1; first list element: List of 1
#> ..$ : num [1:5, 1:2] 107 107 107 107 107 ...
#> ..- attr(*, "class")= chr [1:3] "XY" "POLYGON" "sfg"
#> - attr(*, "sf_column")= chr "geometry"
#> - attr(*, "agr")= Factor w/ 3 levels "constant","aggregate",..: NA NA NA NA NA NA NA NA NA NA ...
#> ..- attr(*, "names")= chr [1:89] "id" "n.patches_Dif" "n.patches_Sig" "n.patches_Slope" ...